Howdy folks! It’s been a long time since I did a coding demonstrations so I thought I’d put one up to provide you a logistic regression example in Python!
Admittedly, this is a cliff notes version, but I hope you’ll get enough from what I have put up here to at least feel comfortable with the mechanics of doing logistic regression in Python (more specifically; using scikit-learn, pandas, etc…). This logistic regression example in Python will be to predict passenger survival using the titanic dataset from Kaggle. Before launching into the code though, let me give you a tiny bit of theory behind logistic regression.
Logistic Regression Formulas:
The logistic regression formula is derived from the standard linear equation for a straight line. As you may recall from grade school, that is y=mx + b . Using the Sigmoid function (shown below), the standard linear formula is transformed to the logistic regression formula (also shown below). This logistic regression function is useful for predicting the class of a binomial target feature.
Logistic Regression Assumptions
Any logistic regression example in Python is incomplete without addressing model assumptions in the analysis. The important assumptions of the logistic regression model include:
- Target variable is binary
- Predictive features are interval (continuous) or categorical
- Features are independent of one another
- Sample size is adequate – Rule of thumb: 50 records per predictor
So, in my logistic regression example in Python, I am going to walk you through how to check these assumptions in our favorite programming language.

Uses for Logistic Regression
One last thing before I give you the logistic regression example in Python / Jupyter Notebook… What awesome result can you ACHIEVE USING LOGISTIC REGRESSION?!? Well, a few things you can do with logistic regression include:
- You can use logistic regression to predict whether a customer will convert (READ: buy or sign-up) to an offer. (will not convert – 0 / will convert – 1)
- You can use logistic regression to predict and preempt customer churn. (will not drop service – 0 / will drop service – 1)
- You can use logistic regression in clinical testing to predict whether a new drug will cure the average patient. (will not cure – 0 / will cure -1)
The nice thing about logistic regression is that it not only predicts an outcome, it also provides a probability of that prediction being correct.
Now For that Logistic Regression Example in Python
That’s it! That’s what I’ve got. I wish I had more time to type up all the information explaining every detail of the code, but well… Actually, that would be redundant. I cover it all right over here on Lynda.com / LinkedIn Learning.
More resources to get ahead...
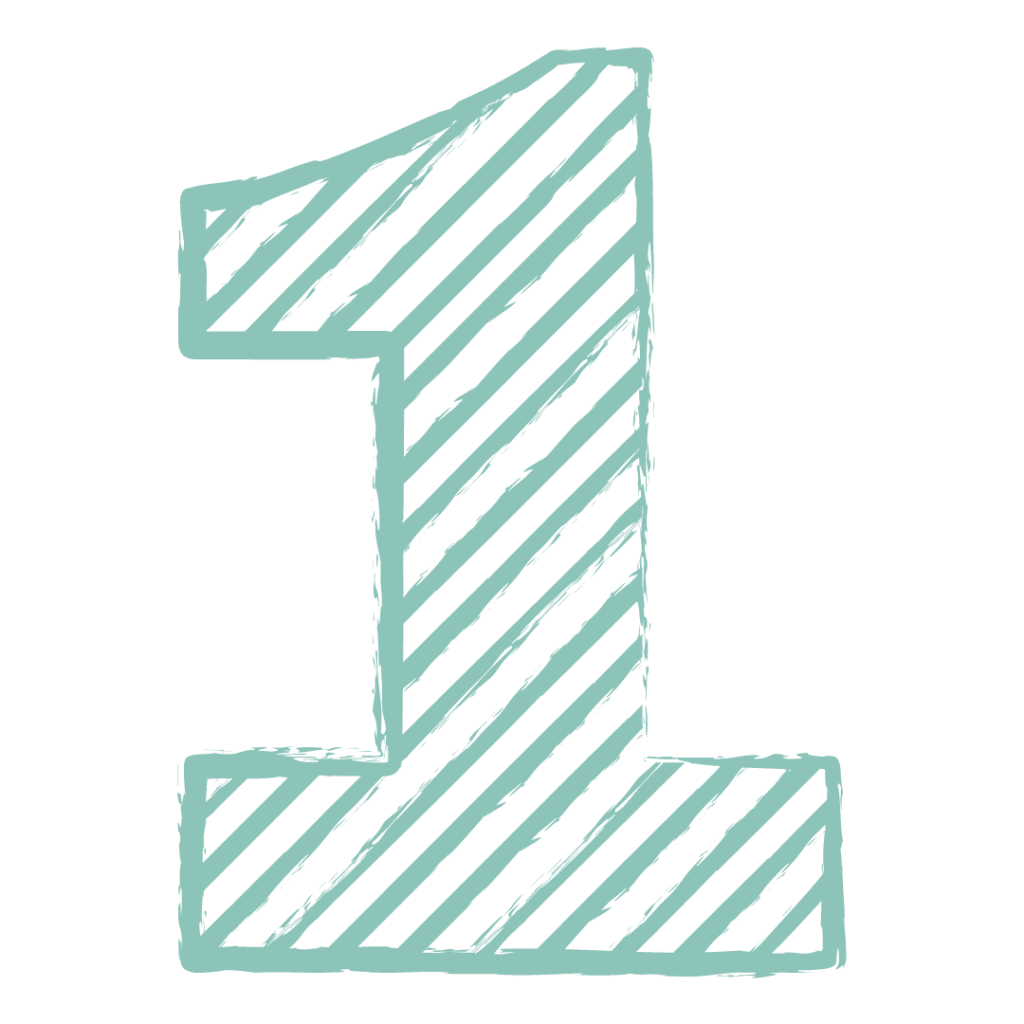
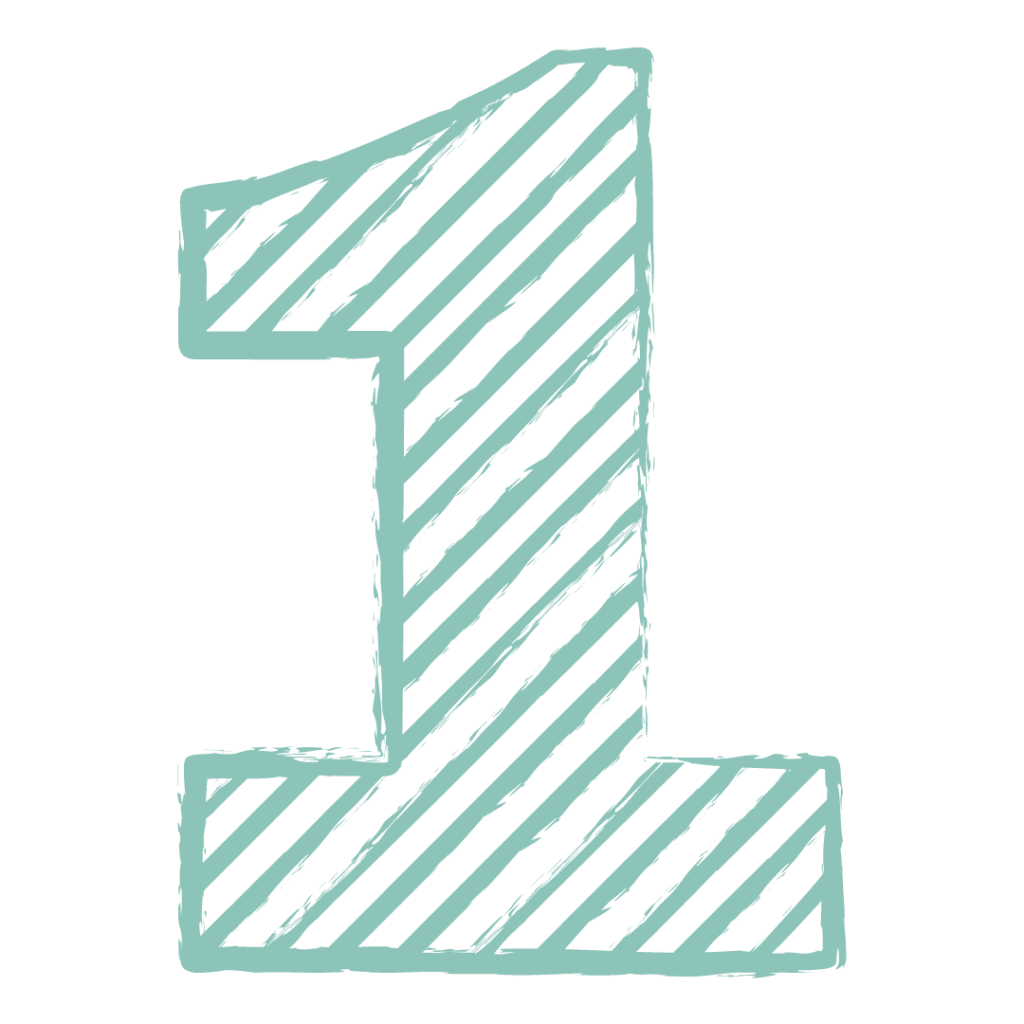
Get Income-Generating Ideas For Data Professionals
Are you tired of relying on one employer for your income? Are you dreaming of a side hustle that won’t put you at risk of getting fired or sued? Well, my friend, you’re in luck.
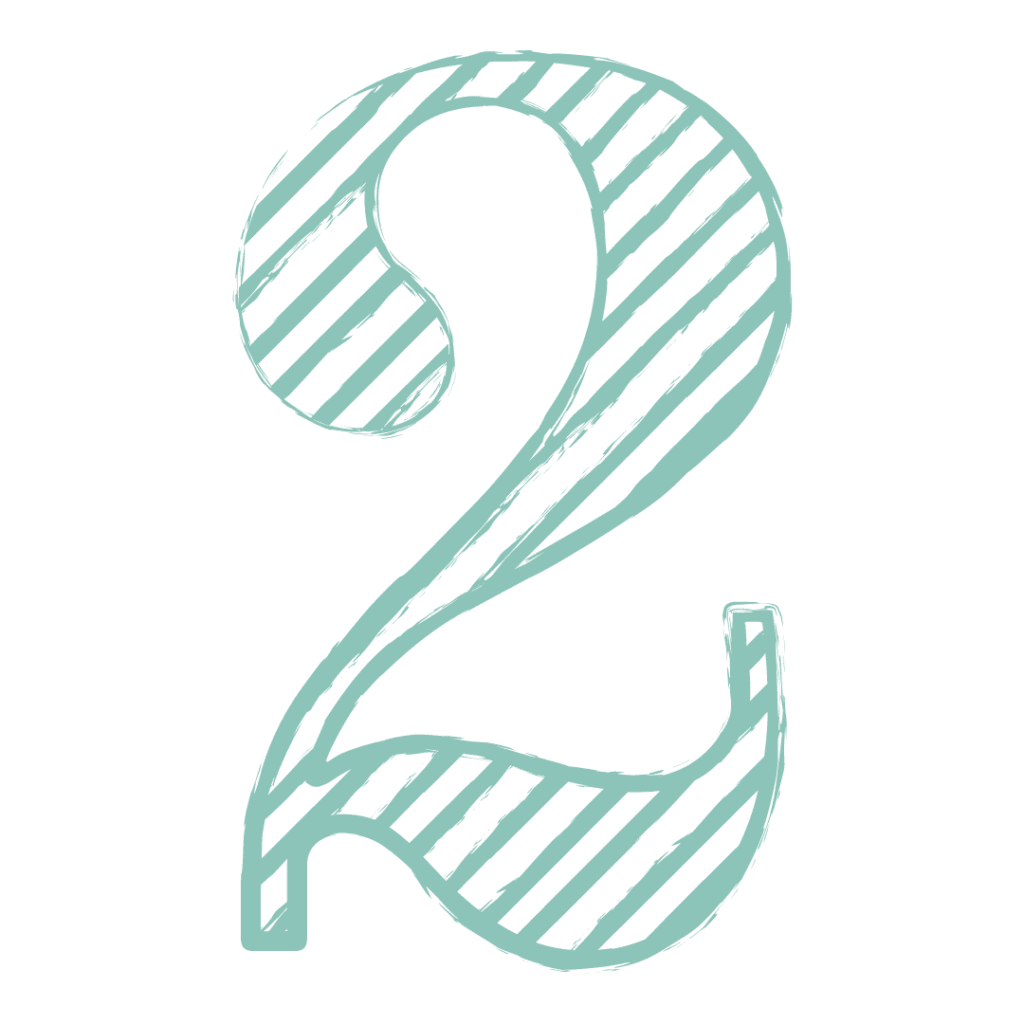
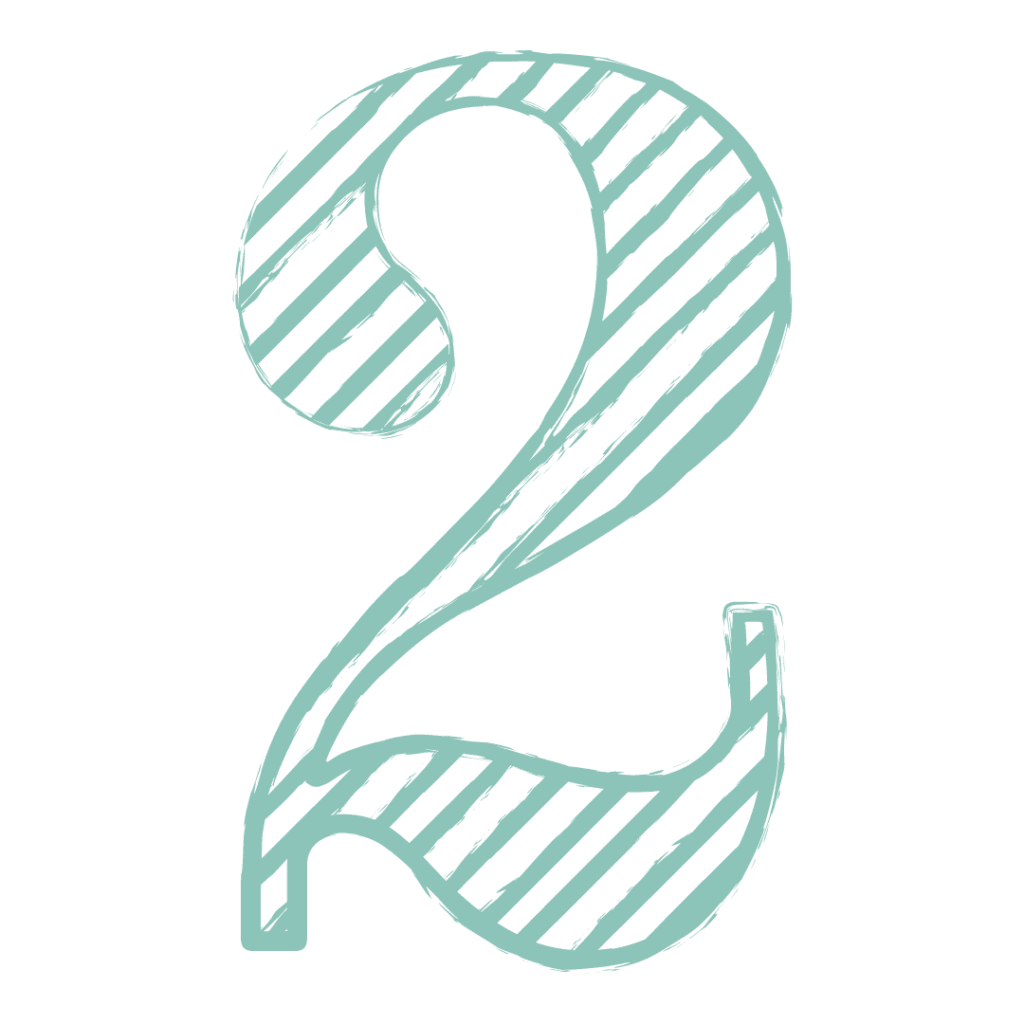