AI vs. ML vs. DL vs. Data Science – How are they different from each other and how are they related? Data professionals work with many technologies that may, at first, seem similar. Adding to the confusion is that some media sources use the terms interchangeably.
AI vs. ML vs. DL vs. Data Science: Learning the Data Lingo Fast!
In this article, we’re going to learn the differences between artificial intelligence (AI) and machine learning (ML) and how does deep learning (DL) relate to those two. Then, we’ll find out how data science fit into all these terms.
Artificial intelligence and machine learning are two much-discussed topics in today’s society. A machine with AI capabilities can make decisions without ongoing human input. Although humans helped with training, they don’t influence the choices made after that point.
Consider the example of an AI fraud detection system used by a credit card company. Humans teach the tool to recognize the most common characteristics of unusual payment activity. However, once it learns those, it can flag suspicious transactions without human help.
Think of AI as an umbrella term related to teaching computers to perform decision-making based on inputted data. AI falls into these three subcategories:
- Artificial Narrow Intelligence/Weak AI: Machines that are good at performing specific, well-defined tasks.
- Artificial General Intelligence/Strong AI: Efforts to make machines have all the capabilities of humans, including consciousness.
- Artificial Superintelligence: Instances where machines surpass human knowledge.
Then, machine learning encompasses the statistical tools that allow extracting insights from the data. There are three machine learning training methods:
- Supervised learning: This machine learning training method concerns previously labeled data. The material consists of training examples in pairs, with an input and the desired output.
- Unsupervised learning: Since unsupervised learning does not use labeled data, the output remains unknown. This learning method relies on mathematics and other techniques to group similar data through a process called clustering.
- Semi-supervised/reinforcement learning: This approach begins with labeled data. However, later in the process, the algorithm also gets exposed to unlabeled material. It then classifies that new content based on things learned from the labeled content.
The Details of ML Vs. DL
You now know that machine learning is a subset of AI. However, perhaps you’re curious about how ML differs from deep learning (DL). For starters, DL is a subset of machine learning.
It uses a multineural network architecture to simulate how the human brain learns things. Like ML, DL has several main training methods:
Convolutional neural networks (CNN): This training type involves multilayered units called a perceptron that detects certain characteristics in the input data. Then, the accompanying convolutional layers create feature maps that record portions of images before breaking them down into rectangular segments for processing. Thus, people commonly choose CNN for picture recognition tasks.
Recurrent neural networks (RNN): A recurrent neural network has nodes that act as memory cells. Thus, it can recall information learned over time and make corrections based on more recent information. Although an RNN eventually becomes self-learning, it requires significant training to reach that point. These networks work best on data related to time series.
The main thing to remember is that DL trains machines or computers in ways that mimic how humans learn. The networks’ structures look similar to the brain and can be quite complex. DL networks can also automatically discover the features used to classify data, provided they receive enough training. However, ML requires that someone give the initial classifications, and it can later learn from those.
We can bring it all together by thinking of ML and DL as the data analysis tools that power an AI application. Additionally, of those two, DL most closely resembles how a human brain works. Machine learning algorithms improve with more exposure to data.
What About Data Science?
There’s some overlap between AI vs. ML vs. DL vs. Data Science. That’s because many data science platforms have AI features, and may include ML and DL algorithms working in the background to get results. However, data science also involves using mathematical approaches, such as statistics and probabilities.
Advances in cloud computing have greatly increased the information that people can access, even without formal data science training. For example, they can log into a cloud-based tool and quickly see the desired information. The need for increased collaboration has also accelerated cloud growth.
People interested in becoming data scientists or expanding their existing data science knowledge can expect to learn about all the main technologies mentioned above — AI, ML and DL. However, they also need a strong foundation in mathematics and may learn some cloud computing, too.
This book is for people who want to develop or advance their careers in the data space. It’s also for people who’d like to become independent consultants or entrepreneurs within the data niche.
A Badass’s Guide to Breaking into Data tells the story of how a young environmental engineer retooled her skill set to become a data scientist, and later turned her passion for data into a thriving business. Paramount to this storyline, the ebook provides 52 pages of free advice about how to break into the data professions by either getting a data job or starting your own small consultancy. This book is free to download and share, but please implement its suggestions responsibly.
A Useful Mental Image
An IBM employee published a blog using a nesting doll analogy for most of the concepts explored here. Visualizing it can help solidify them for you. Think of AI as the biggest doll, then ML as the medium-sized one, and DL as the smallest of the three. That’s because each of these is a component of the technology before it.
The IBM representative also suggested a neural networks nesting doll as one situated between ML and DL. They likely made that distinction because not all neural networks get used for DL tasks. A neural network should have at least three layers to get categorized as one used for deep learning.
Using the Terminology With Confidence
This explainer about AI vs. ML vs. DL vs. Data Science should dispel the confusion that many people feel when they see these common technologies and wonder about the specifics between them. Then, they can feel more confident whether learning more about these advancements or discussing them with others.
A Guest Post By...
This blog post was generously contributed to Data-Mania by Shannon Flynn. Shannon Flynn is a freelance blogger who covers business, cybersecurity and IoT topics.
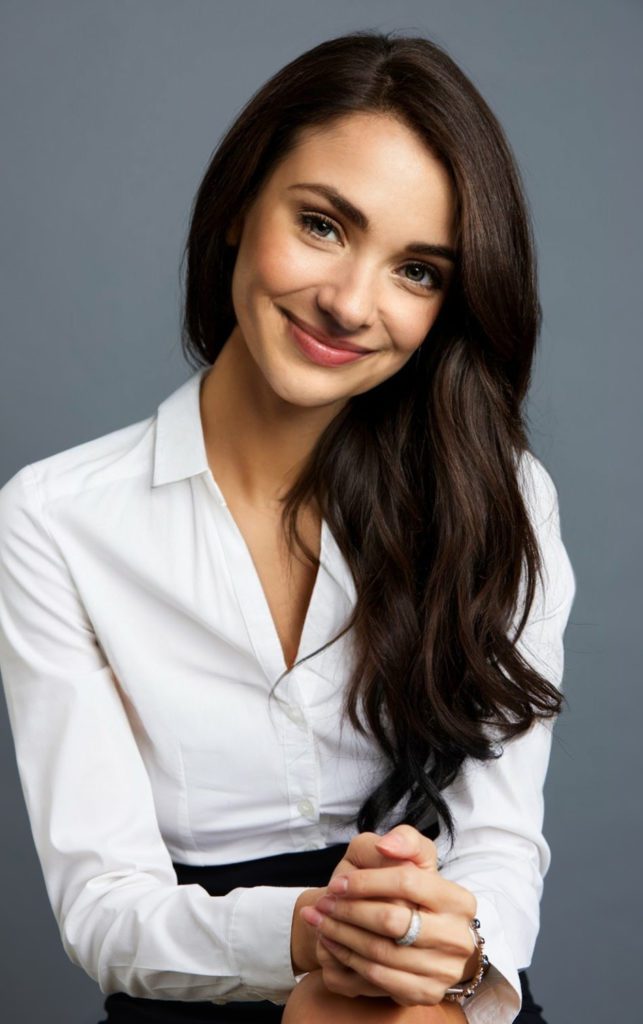
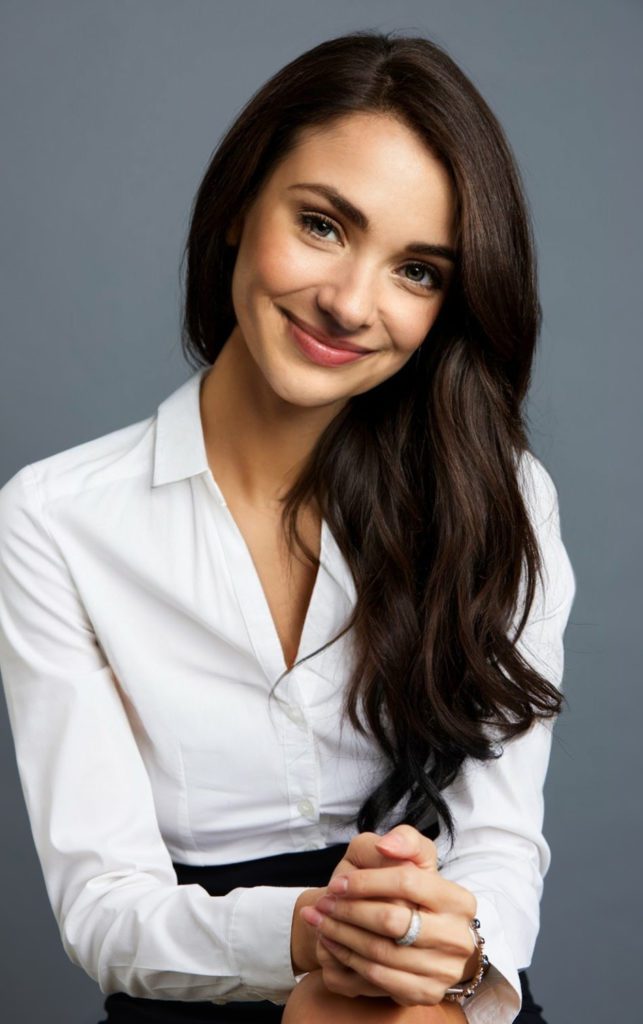