If you’re considering learning Machine Learning (ML), read these top 4 reasons NOT to learn machine learning.
With all the ML hype out there these days, all you ever hear about is how machine learning and data scientists are so great. No one ever talks about the drawbacks of becoming a data scientist. So, in today’s update I’m going to give you a data science insider’s take on the top 4 reasons not to spend too much time learning machine learning, especially in 2021.
YouTube URL: https://youtu.be/kkqav8RwCiQ
If you prefer to read instead of watch then, read on…
Learn Machine Learning – Why Am I Qualified to Give You Advice
As far as why I’m qualified to give advice on machine learning, I’ve been in the data science field full time since 2012. And I’ve educated over 1.3 million people on machine learning, data science, and topics on data strategy. So, I know a thing or two about machine learning.
If you’re new around here… Hi, I’m Lillian Pierson and I support data professionals to become world-class data leaders and entrepreneurs.
Don’t worry, this isn’t a gripe session on Machine Learning (ML) or Data Science (DS), nor is it click-bait… with each other 4 reasons, I’ll be providing clear, evidence-based explanations of what the problem is and why it matters to data professionals… I’ll be providing solutions for how to avoid these problems in the first place.
Is it Good to Learn Machine Learning?
Let me also preface the discussion with a clear statement of all the reasons it’s good to learn machine learning, especially in 2021.
- The limitless career opportunities if you know ML – both as an employee, freelancer or business owner.
- Tons of free and low-cost online training courses.
- If you have a STEM degree, learning ML is almost a walk in the park.
- One day, data literacy will be a fundamental requirement for all knowledge workers. If you want to work in the tech industry, then expect to be required to at least know how ML works, period.
I am not saying that learning machine learning is a bad idea. In fact, it’s one of the smartest choices I ever made. What I am about to share with you are the less than desirable facts about learning machine learning that no one ever tells you.
Top 4 Reasons NOT to Learn Machine Learning
The following are some good reasons you should look into as to why you should not learn Machine Learning (ML):
1. It takes a really long time to get good at machine learning
WHAT: Machine learning is super complicated. Not only do you have to be a good coder or developer, you also have to be pretty sophisticated with applied Mathematics and Statistics.
WHY: It’s easy to work through courses and learn the basics of machine learning algorithms, but actually bringing them into production and applying those to solve business problems as a whole – it’s a whole other thing.
Just like programming and being a software engineer, you could just spend – I’ve seen guys that invented deep learning libraries and then twenty to twenty-five years they’d say, – I’m still not a data science expert, I’m still not a machine learning expert. It’s competitive and it takes a really long time to get good.
THE SOLUTION: The solution to this then is not if you say – OK, I want to learn machine learning, I’m committed and ready to go the long haul – you can get in there and be like, OK this is intense. You can still keep that same expertise and apply that expertise to create value in different ways beyond just coding. So, you have to really start early.
Look at what your calling is within your career within the data space. If you want to be an implementation person and you want to be building solutions, then just expect it to take several years.
But if you want to be a leader, a product manager, an entrepreneur, you need to spend a decade getting good at machine learning in order to make that happen. Do you need to know machine learning? Yes, that’s a good idea – you need to get the basics covered, but you don’t need to be an expert at machine learning in order to become an expert in other areas of the data profession and just data science overall.
2. It is nearly impossible to land a job as a data scientist
WHAT: You could go into a whole coding course or get a data science degree, all of that and you still may not be able to land a job as a data scientist.
WHY: The obvious thing is that most people think that – OK, I go to school, I learn something today and I can get a job and make money. But in the data science field, it doesn’t really work out that way, unfortunately.
So, people look at these data science degrees and then they end up training, because just having machine learning skills doesn’t get you a job as a data scientist.
THE SOLUTION: “Data science” degrees – can’t land a job. Just don’t expect that you’re going to be a data scientist if you learn machine learning. If you already have a STEM degree, you don’t need to go get another degree in data science. Because data science really, if you look at Drew Conaway’s definition of data science, one third of it is subject matter expertise. It doesn’t really make sense.
If you don’t have a STEM degree, then maybe getting a job in data science is your segue into working as a data scientist or working in the field of data science. Steer clear of data science degrees unless you don’t have a STEM degree.
3. Implementing ML isn’t all that “sexy”
WHAT: Coding and data science implementation work is not at all that “sexy”. So back in 2012, Harvard came out with “data scientist is the sexiest job of the 21st century”. It’s cool, it’s a powerful skill set. Machine learning and AI are definitely changing the world and I definitely want to be a part of that.
WHY: You have to look at your personality. It all comes back really to leadership and the different types of personality.
So, some people want to make an impact and they feel called to make a positive impact on the world through technology. They don’t want to deal with people. Then others want to make a positive impact on the world but we feel led to make an impact on people. If you’re like me, and I’m more drawn to people and helping people, then getting a job implementing machine learning is not going to make you happy.
If you’re drawn to helping people, machine learning probably isn’t for you because it’s not going to satisfy your inner need where you feel like you’re satisfied that you helped someone. Implementing machine learning for people like me is not sexy. But for people, like my husband, who love to code, they don’t want to deal with people, they don’t want to build a business, they don’t want to manage a team, they want to build solutions – and it’s sexy – make loads of money and just with machine learning models all day.
THE SOLUTION: The solution is looking at what you want to do, and what your calling is. And you need to learn machine learning, have your end goal in mind like “what are you actually going to do with it?”
Learning machine learning doesn’t necessarily mean you’re going to be a data scientist in implementing data science all the time. It may mean you’re going to, like what I did, I learned data science, I learned machine learning. And then I educated people. I trained people around the world, I did data strategy, and I impacted businesses by impacting people. So, it just depends on your personality.
4. Just Learning ML may not get you where you want to go
ML is only a very small part of these roles, if it has a place at all:
- if you want to consult with people in the business;
- if you want to vision products and take them to market; and,
- if you want to use data to help you create better products and services.
WHAT: This is really a re-emphasis or it builds on the earlier points of that. I assume that if you’re learning machine learning, you want to get a job in data science. You think you’re going to be a data scientist. You think you’re going to enjoy that because it’s the sexiest job of the 21st century. But any one of those three things could be wrong.
WHY: So, one could learn machine learning and then end up working like hedge funds where they have you working 60 to 80 hours a week. And you know, it’s high pressure and you definitely cannot mess up, and you get paid a lot of money.
But people are just not happy and it’s not nice seeing people do that. And they’re like, wow, this money cannot buy back happiness by doing this. Thinking of reverse engineering, you have to figure it out yourself. It’s like tapping into who you are as a person and figuring out. What is the impact I want to have with my life? And what lifestyle do I want to live and how do I want to change the world?
Then find opportunities and rules that act as a vehicle. Use it as a pathway by which you can do those things, and have those things. Once you’ve identified what that is, figure out the expertise and what you need in order to get there.
Now chances are if it’s in the data space, learning machine learning is going to be a part of that. But becoming a data machine learning expert and implementing for 15 years, not necessarily what’s going to be on the bill of other types of data science.
THE SOLUTION: Just listen to your heart. It starts with a goal in mind and then try to figure out you want to learn machine learning. But how much do you need to know? And just keep a pulse on that.
People who want to make an impact through technology are implementation people. People that want to make an impact through people – they’re usually leaders or maybe entrepreneurs.
Those are the real three archetypes of data professionals I see out there. What I would say about machine learning – go ahead and learn it.
Conclusion
I love machine learning. I even wrote a book about it called Data Science for Dummies. However, I understand why people are hesitant to take on the subject of machine learning. If you’re like most people that don’t have a lot of time – then you don’t want to invest a lot of time in something that might not lead to a permanent job. If you’re thinking about taking up machine learning, I’d recommend starting with an end goal in sight.
More on how to go about doing that in: http://data-mania.com/blog/how-long-does-it-take-to-learn-how-to-code/
More to Know…
If you like this topic on machine learning, and you think you might have some interest in reasons why you don’t want to learn it, then you’d probably really like the video I created on Do the 4 Types of Analytics Even Matter in 2021 where I talked about the four different types of analytics and why they may or may not matter anymore in 2021.
Hey! If you liked this post, I’d really appreciate it if you’d share the love by clicking on one of the share buttons below!
More free resources that'll help...
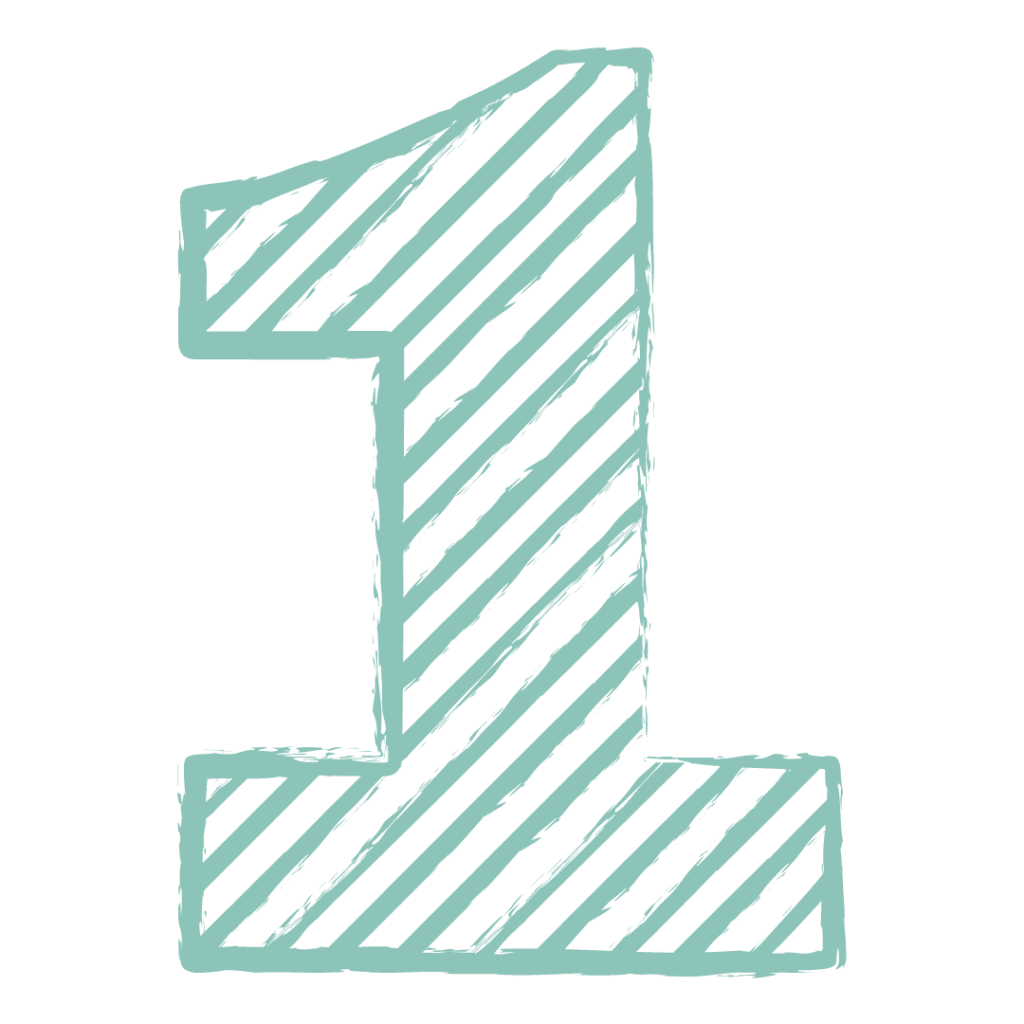
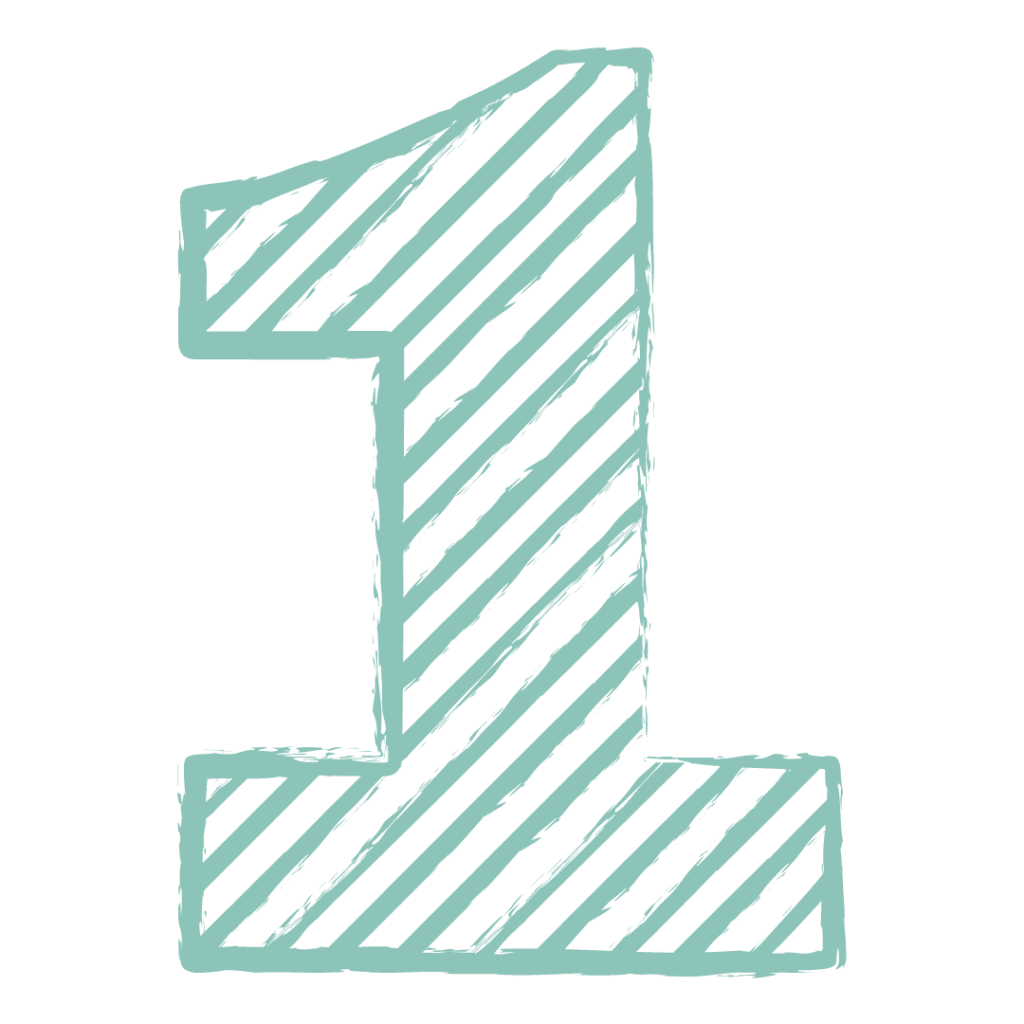
Get The Badass's Guide To Breaking Into Data
I was working a 9-to-5 as a data analytics developer back in 2012 when I started Data-Mania. With that transition, the seed was planted to write an ebook that helps other people break into the field that'd been so generous to me. You can’t keep something like this to yourself, right? 😉 Today we’ve published this free ebook, and it's helped thousands of people just like you make the transition....
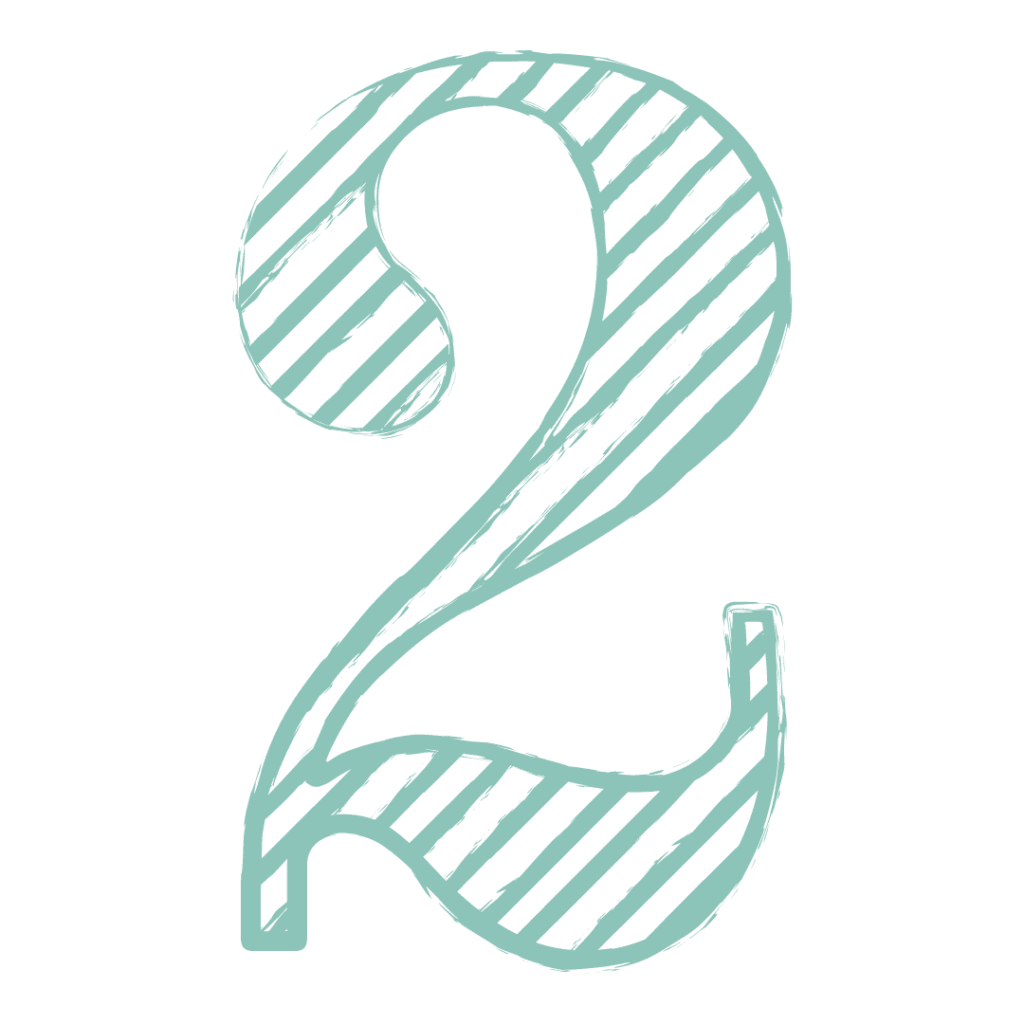
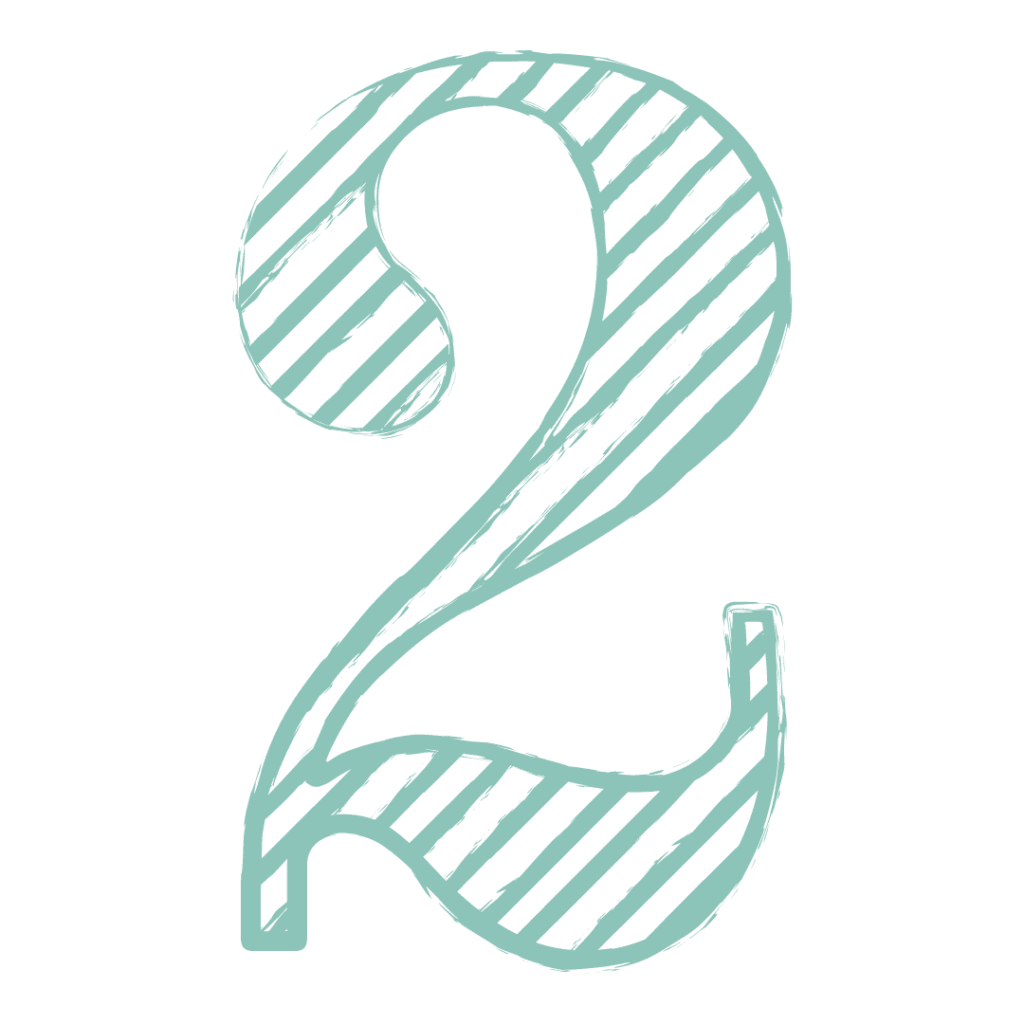
Take The Data Superhero Quiz
You can take a much more direct path to the top once you understand how to leverage your skillsets, your talents, your personality and your passions in order to serve in a capacity where you’ll thrive. That’s why I’m encouraging you to take the data superhero quiz.
NOTE: This description contains affiliate links. They allow you to find the items mentioned in this video and support the channel at no cost to you. While this channel may earn minimal sums when the viewer uses the links, the viewer is in NO WAY obligated to use these links. Thank you for your support!